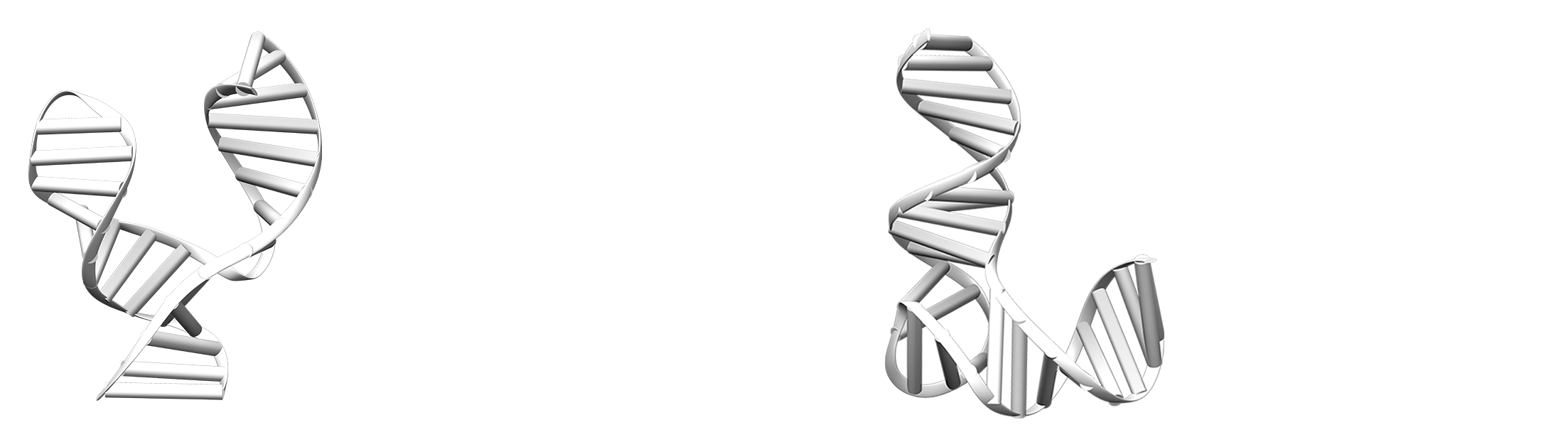
Journal of Chemical Information and Modeling DOI: 10.1021/acs.jcim.4c02441 Published: 2025-03-27
Machine learning potentials (MLPs) have revolutionized molecular simulation by providing efficient and accurate models for predicting atomic interactions. MLPs continue to advance and have had profound impact in applications that include drug discovery, enzyme catalysis, and materials design. The current landscape of MLP software presents challenges due to the limited interoperability between packages, which can lead to inconsistent benchmarking practices and necessitates separate interfaces with molecular dynamics (MD) software. To address these issues, we present DeePMD-GNN, a plugin for the DeePMD-kit framework that extends its capabilities to support external graph neural network (GNN) potentials.DeePMD-GNN enables the seamless integration of popular GNN-based models, such as NequIP and MACE, within the DeePMD-kit ecosystem. Furthermore, the new software infrastructure allows GNN models to be used within combined quantum mechanical/molecular mechanical (QM/MM) applications using the range corrected ΔMLP formalism.We demonstrate the application of DeePMD-GNN by performing benchmark calculations of NequIP, MACE, and DPA-2 models developed under consistent training conditions to ensure fair comparison.