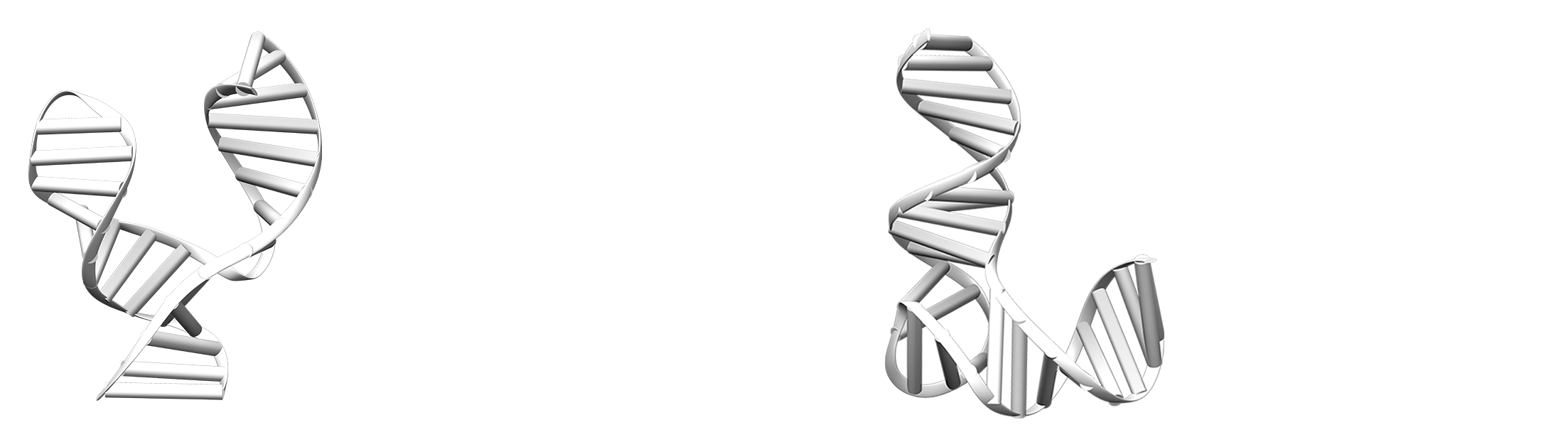
vol. 1397 p. 161-204 DOI: 10.1021/bk-2021-1397.ch007 ISBN: 9780841298057 Published: 2021-11-19
Recent concurrent advances in methodology development, computer hardware and simulation software has transformed our ability to make practical, quantitative predictions of relative ligand binding affinities to guide rational drug design. In the past, these calculations have been hampered by the lack of affordable software with highly efficient implementations of state-of-the-art methods on specialized hardware such as graphical processing units, combined with the paucity of available workflows to streamline throughput for real-world industry applications. Herein we discuss recent methodology development, GPU-accelerated implementation, and workflow creation for alchemical free energy simulation methods in the AMBER Drug Discovery Boost (AMBER-DD Boost) package available as a patch to AMBER20. Among the methodological advances are 1) new methods for the treatment of softcore potentials that overcome long standing end-point catastrophe and softcore imbalance problems and enable single-step alchemical transformations between ligands, 2) new adaptive enhanced sampling methods in the ”alchemical” (or ” λ”) dimension to accelerate convergence and obtain high precision ligand binding affinity predictions, 3) robust network-wide analysis methods that include cycle closure and reference constraints and restraints, and 4) practical workflows that enable streamlined calculations on large datasets to be performed. Benchmark calculations on various systems demonstrate that these tools deliver an outstanding combination of accuracy and performance, resulting in reliable high-throughput binding affinity predictions at affordable cost.