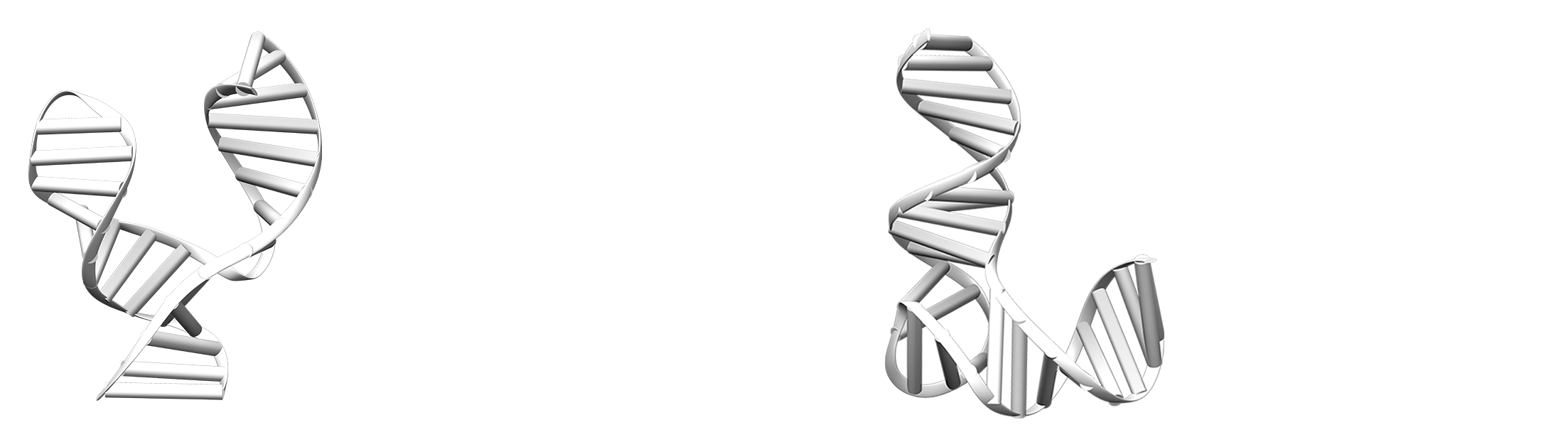
DOI: 10.1063/9780735425279_006 ISBN: 978-0-7354-2527-9 Published: 2023-01-26
A new direction has emerged in molecular simulations in recent years, where potential energy surfaces (PES) are constructed using machine learning (ML) methods. These ML models, combining the accuracy of quantum mechanical models and the efficiency of empirical atomic potential models, have been demonstrated by many studies to have extensive application prospects. This chapter introduces a recently developed ML model, Deep Potential (DP), and the corresponding package, DeePMD-kit. First, we present the basic theory of the DP method. Then, we show how to train and test a DP model for a gas-phase methane molecule using the DeePMD-kit package. Next, we introduce some recent progress on simulations of biomolecular processes by integrating the DeePMD-kit with the AMBER molecular simulation software suite. Finally, we provide a supplement on points that require further explanation.